Got this from ChatGPT and tested.
Go to apps script:
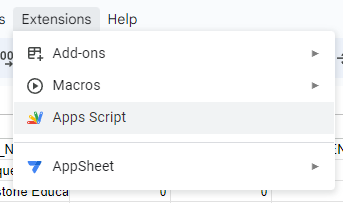
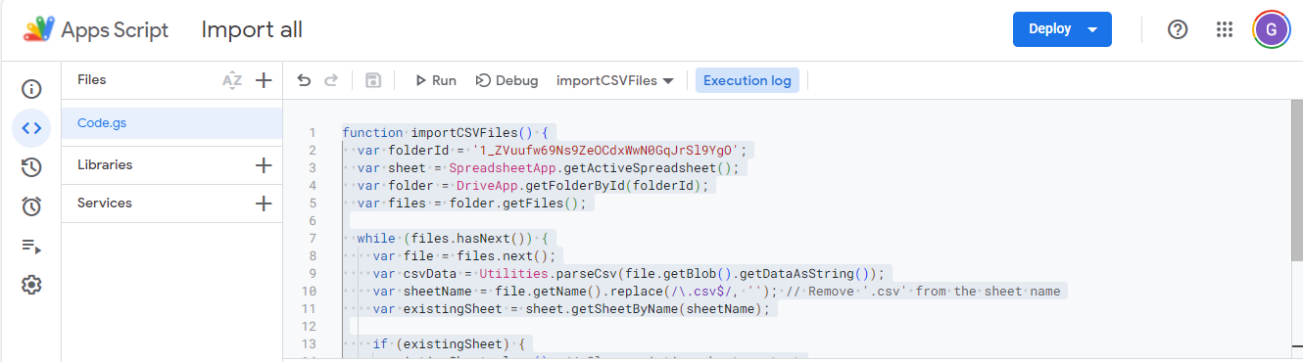
Paste the script below, add your folder ID, and save. The folder ID can be obtained from the URL to the folder:
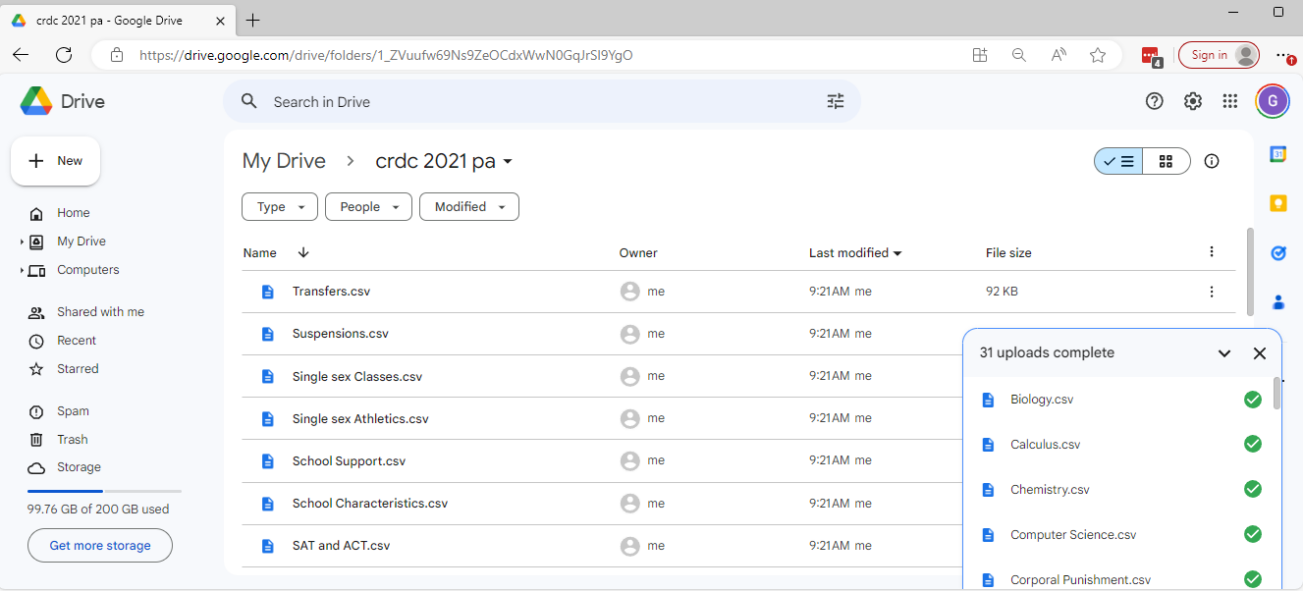
Then, click Run – you will have to go through several security prompts to agree to trust the script.
function importCSVFiles() {
var folderId = '1_ZVuufw69Ns9ZeOCdxWwN0GqJrSl9YgO';
var sheet = SpreadsheetApp.getActiveSpreadsheet();
var folder = DriveApp.getFolderById(folderId);
var files = folder.getFiles();
while (files.hasNext()) {
var file = files.next();
var csvData = Utilities.parseCsv(file.getBlob().getDataAsString());
var sheetName = file.getName().replace(/\.csv$/, ''); // Remove '.csv' from the sheet name
var existingSheet = sheet.getSheetByName(sheetName);
if (existingSheet) {
existingSheet.clear(); // Clear existing sheet content
} else {
sheet.insertSheet(sheetName); // Create a new sheet if it doesn't exist
}
var targetSheet = sheet.getSheetByName(sheetName);
targetSheet.getRange(1, 1, csvData.length, csvData[0].length).setValues(csvData);
}
}